Python-Markdown provides two public functions (markdown and markdownFromFile) both of which wrap the public class Markdown.If you’re processing one document at a time, the functions will serve your needs. However, if you need to process multiple documents, it may be advantageous to create a single instance of the class: ` Markdown class and pass multiple documents through it. This script turns Markdown into HTML using the Python markdown library and wraps the result in a complete HTML document with default Bootstrap styling so that it's immediately printable. Requires the python libraries jinja2, markdown, and mdxsmartypants. With the md-in-html extension enabled, the content of a raw HTML block-level element can be parsed as Markdown by including a markdown attribute on the opening tag. The markdown attribute will be stripped from the output, while all other attributes will be preserved. The markdown attribute can be assigned one of three values: '1', 'block', or 'span'. Convert Markdown to HTML in Python The easiest way to convert is just use a string for input and a string for output. Import markdown # Simple conversion in memory mdtext = '# Hellonn.Text.' html = markdown.markdown(mdtext) print(html) To use files for input and output instead.
As developers, we rely on static analysis tools to check, lint and transform our code. We use these tools to help us be more productive and produce better code. However, when we write content using markdown the tools at our disposal are scarce.
In this article we describe how we developed a Markdown extension to address challenges in managing content using Markdown in Django sites.
- The Problem
- Using Markdown
- Validate and Transform Django Links
- Handling Internal and External Links
- Conclusion
The Problem
Like every website, we have different types of (mostly) static content in places like our home page, FAQ section and 'About' page. For a very long time, we managed all of this content directly in Django templates.
When we finally decided it's time to move this content out of templates and into the database, we thought it's best to use Markdown. It's safer to produce HTML from Markdown, it provides a certain level of control and uniformity, and is easier for non-technical users to handle. As we progressed with the move, we noticed we are missing a few things:
Internal Links
Links to internal pages can get broken when the URL changes. In Django templates and views we use reverse
and {% url %}
, but this is not available in plain Markdown.
Copy Between Environments
Absolute internal links cannot be copied between environments. This can be resolved using relative links, but there is no way to enforce this out of the box.
Invalid Links
Invalid links can harm user experience and cause the user to question the reliability of the entire content. This is not something that is unique to Markdown, but HTML templates are maintained by developers who know a thing or two about URLs. Markdown documents on the other hand, are intended for non-technical writers.
Prior Work
When I was researching this issue I searched for Python linters, Markdown preprocessor and extensions to help produce better Markdown. I found very few results. One approach that stood out was to use Django templates to produce Markdown documents.
Preprocess Markdown using Django Template
Using Django templates, you can use template tags such as url
to reverse URL names, as well as conditions, variables, date formats and all the other Django template features. This approach essentially uses Django template as a preprocessor for Markdown documents.
I personally felt like this may no be the best solution for non-technical writers. In addition, I was worried that providing access to Django template tags might be dangerous.
Using Markdown
With a better understanding of the problem, we were ready to dig a bit deeper into Markdown in Python.
Converting Markdown to HTML
To start using Markdown in Python, install the markdown
package:
Next, create a Markdown
object and use the function convert
to turn some Markdown into HTML:
You can now use this HTML snippet in your template.

Using Markdown Extensions
The basic Markdown processor provides the essentials for producing HTML content. For more 'exotic' options, the Python markdown
package includes some built-in extensions. A popular extension is the 'extra' extension that adds, among other things, support for fenced code blocks:
To extend Markdown with our unique Django capabilities, we are going to develop an extension of our own.
Creating a Markdown Extension to Process Inline Links
Python Markdown Example
If you look at the source, you'll see that to convert markdown to HTML, Markdown
uses different processors. One type of processor is an inline processor. Inline processors match specific inline patterns such as links, backticks, bold text and underlined text, and converts them to HTML.
The main purpose of our Markdown extension is to validate and transform links. So, the inline processor we are most interested in is the LinkInlineProcessor
. This processor takes markdown in the form of [Haki's website](https://hakibenita.com)
, parses it and returns a tuple containing the link and the text.
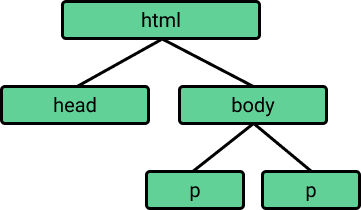
To extend the functionality, we extend LinkInlineProcessor
and create a Markdown.Extension
that uses it to handle links:
Let's break it down:
- The extension
DjangoUrlExtension
registers an inline link processor calledDjangoLinkInlineProcessor
. This processor will replace any other existing link processor. - The inline processor
DjangoLinkInlineProcessor
extends the built-inLinkInlineProcessor
, and calls the functionclean_link
on every link it processes. - The function
clean_link
receives a link and a domain, and returns a transformed link. This is where we are going to plug in our implementation.
How to get the site domain
To identify links to your own site you must know the domain of your site. If you are using Django's sites framework you can use it to get the current domain.
I did not include this in my implementation because we don't use the sites framework. Instead, we set a variable in Django settings.
Another way to get the current domain is from an HttpRequest
object. If content is only edited in your own site, you can try to plug the site domain from the request object. This may require some changes to the implementation.
To use the extension, add it when you initialize a new Markdown
instance:
Great, the extension is being used and we are ready for the interesting part!
Validate and Transform Django Links
Now that we got the extension to call clean_link
on all links, we can implement our validation and transformation logic.
Validating mailto
Links
To get the ball rolling, we'll start with a simple validation. mailto
links are useful for opening the user's email client with a predefined recipient address, subject and even message body.
A common mailto
link can look like this:
This link will open your email client set to compose a new email to 'support@service.com' with subject line 'I need help!'.
mailto
links do not have to include an email address. If you look at the 'share' buttons at the bottom of this article, you'll find a mailto
link that looks like this:
Markdown Import File
This mailto
link does not include a recipient, just a subject line and message body.
Now that we have a good understanding of what mailto
links look like, we can add the first validation to the clean_link
function:
To validate a mailto
link we added the following code to clean_link
:
- Check if the link starts with
mailto:
to identify relevant links. - Split the link to its components using a regular expression.
- Yank the actual email address from the
mailto
link, and validate it using Django'sEmailValidator
.
Notice that we also added a new type of exception called InvalidMarkdown
. We defined our own custom Exception
type to distinguish it from other errors raised by markdown
itself.
Custom error class
I wrote about custom error classes in the past, why they are useful and when you should use them.
Before we move on, let's add some tests and see this in action:
Great! Worked as expected.
Handling Internal and External Links
Now that we got our toes wet with mailto
links, we can handle other types of links:
External Links
- Links outside our Django app.
- Must contains a scheme: either http or https.
- Ideally, we also want to make sure these links are not broken, but we won't do that now.
Internal Links
- Links to pages inside our Django app.
- Link must be relative: this will allow us to move content between environments.
- Use Django's URL names instead of a URL path: this will allow us to safely move views around without worrying about broken links in markdown content.
- Links may contain query parameters (
?
) and a fragment (#
).
SEO
From an SEO standpoint, public URL's should not change. When they do, you should handle it properly with redirects, otherwise you might get penalized by search engines.
With this list of requirements we can start working.
Resolving URL Names
To link to internal pages we want writers to provide a URL name, not a URL path. For example, say we have this view:
The URL path to this page is https://example.com/
, the URL name is home
. We want to use the URL name home
in our markdown links, like this:
This should render to:
We also want to support query params and hash:
This should render to the following HTML:
Using URL names, if we change the URL path, the links in the content will not be broken. To check if the href provided by the writer is a valid url_name
, we can try to reverse
it:
The URL name 'home' points to the url path '/'. When there is no match, an exception is raised:
Before we move forward, what happens when the URL name include query params or a hash:
This makes sense because query parameters and hash are not part of the URL name.
To use reverse
and support query params and hashes, we first need to clean the value. Then, check that it is a valid URL name and return the URL path including the query params and hash, if provided:
This snippet uses a regular expression to split href
in the occurrence of either ?
or #
, and return the parts.
Make sure that it works:
Amazing! Writers can now use URL names in Markdown. They can also include query parameters and fragment to be added to the URL.
Handling External Links
To handle external links properly we want to check two things:
- External links always provide a scheme, either
http:
orhttps:
. - Prevent absolute links to our own site. Internal links should use URL names.
So far, we handled URL names and mailto
links. If we passed these two checks it means href
is a URL. Let's start by checking if the link is to our own site:
The function urlparse
returns a named tuple that contains the different parts of the URL. If the netloc
property equals the site_domain
, the link is really an internal link.
If the URL is in fact internal, we need to fail. But, keep in mind that writers are not necessarily technical people, so we want to help them out a bit and provide a useful error message. We require that internal links use a URL name and not a URL path, so it's best to let writers know what is the URL name for the path they provided.
To get the URL name of a URL path, Django provides a function called resolve
:
When a match is found, resolve
returns a ResolverMatch
object that contains, among other information, the URL name. When a match is not found, it raises an error:
This is actually what Django does under the hood to determine which view function to execute when a new request comes in.
To provide writers with better error messages we can use the URL name from the ResolverMatch
object:
When we identify that the link in internal, we handle two cases:
- We don't recognize the URL: The url is most likely incorrect. Ask the writer to check the URL for mistakes.
- We recognize the URL: The url is correct so tell the writer what URL name to use instead.
Let's see it in action:
Nice! External links are accepted and internal links are rejected with a helpful message.
Requiring Scheme
The last thing we want to do is to make sure external links include a scheme, either http:
or https:
. Let's add that last piece to the function clean_link
:
Using the parsed URL we can easily check the scheme. Let's make sure it's working:
We provided the function with a link that has no scheme, and it failed with a helpful message. Cool!
Putting it All Together
Python Import Markdown File
This is the complete code for the clean_link
function:
To get a sense of what a real use case for all of these features look like, take a look at the following content:
This will produce the following HTML:
Nice!
Conclusion
We now have a pretty sweet extension that can validate and transform links in Markdown documents! It is now much easier to move documents between environments and keep our content tidy and most importantly, correct and up to date!
Source
The full source code can be found in this gist.
Taking it Further
The capabilities described in this article worked well for us, but you might want to adjust it to fit your own needs.
If you need some ideas, then in addition to this extension we also created a markdown Preprocessor that lets writers use constants in Markdown. For example, we defined a constant called SUPPORT_EMAIL
, and we use it like this:
The preprocessor will replace the string $SUPPORT_EMAIL
with the text we defined, and only then render the Markdown.
In this tutorial, you learn how to convert Juptyer notebooks into Python scripts to make it testing and automation friendly using the MLOpsPython code template and Azure Machine Learning. Typically, this process is used to take experimentation / training code from a Juptyer notebook and convert it into Python scripts. Those scripts can then be used testing and CI/CD automation in your production environment.
A machine learning project requires experimentation where hypotheses are tested with agile tools like Jupyter Notebook using real datasets. Once the model is ready for production, the model code should be placed in a production code repository. In some cases, the model code must be converted to Python scripts to be placed in the production code repository. This tutorial covers a recommended approach on how to export experimentation code to Python scripts.
In this tutorial, you learn how to:
- Clean nonessential code
- Refactor Jupyter Notebook code into functions
- Create Python scripts for related tasks
- Create unit tests
Prerequisites
- Generate the MLOpsPython templateand use the
experimentation/Diabetes Ridge Regression Training.ipynb
andexperimentation/Diabetes Ridge Regression Scoring.ipynb
notebooks. These notebooks are used as an example of converting from experimentation to production. You can find these notebooks at https://github.com/microsoft/MLOpsPython/tree/master/experimentation. - Install
nbconvert
. Follow only the installation instructions under section Installing nbconvert on the Installation page.
Remove all nonessential code
Some code written during experimentation is only intended for exploratory purposes. Therefore, the first step to convert experimental code into production code is to remove this nonessential code. Removing nonessential code will also make the code more maintainable. In this section, you'll remove code from the experimentation/Diabetes Ridge Regression Training.ipynb
notebook. The statements printing the shape of X
and y
and the cell calling features.describe
are just for data exploration and can be removed. After removing nonessential code, experimentation/Diabetes Ridge Regression Training.ipynb
should look like the following code without markdown:
Refactor code into functions
Second, the Jupyter code needs to be refactored into functions. Refactoring code into functions makes unit testing easier and makes the code more maintainable. In this section, you'll refactor:
- The Diabetes Ridge Regression Training notebook(
experimentation/Diabetes Ridge Regression Training.ipynb
) - The Diabetes Ridge Regression Scoring notebook(
experimentation/Diabetes Ridge Regression Scoring.ipynb
)
Refactor Diabetes Ridge Regression Training notebook into functions
In experimentation/Diabetes Ridge Regression Training.ipynb
, complete the following steps:
Create a function called
split_data
to split the data frame into test and train data. The function should take the dataframedf
as a parameter, and return a dictionary containing the keystrain
andtest
.Move the code under the Split Data into Training and Validation Sets heading into the
split_data
function and modify it to return thedata
object.Create a function called
train_model
, which takes the parametersdata
andargs
and returns a trained model.Move the code under the heading Training Model on Training Set into the
train_model
function and modify it to return thereg_model
object. Remove theargs
dictionary, the values will come from theargs
parameter.Create a function called
get_model_metrics
, which takes parametersreg_model
anddata
, and evaluates the model then returns a dictionary of metrics for the trained model.Move the code under the Validate Model on Validation Set heading into the
get_model_metrics
function and modify it to return themetrics
object.
The three functions should be as follows:
Still in experimentation/Diabetes Ridge Regression Training.ipynb
, complete the following steps:
Create a new function called
main
, which takes no parameters and returns nothing.Move the code under the 'Load Data' heading into the
main
function.Add invocations for the newly written functions into the
main
function:Move the code under the 'Save Model' heading into the
main
function.
The main
function should look like the following code:
At this stage, there should be no code remaining in the notebook that isn't in a function, other than import statements in the first cell.
Add a statement that calls the main
function.
After refactoring, experimentation/Diabetes Ridge Regression Training.ipynb
should look like the following code without the markdown:
Refactor Diabetes Ridge Regression Scoring notebook into functions
In experimentation/Diabetes Ridge Regression Scoring.ipynb
, complete the following steps:
- Create a new function called
init
, which takes no parameters and return nothing. - Copy the code under the 'Load Model' heading into the
init
function.
The init
function should look like the following code:
Once the init
function has been created, replace all the code under the heading 'Load Model' with a single call to init
as follows:
In experimentation/Diabetes Ridge Regression Scoring.ipynb
, complete the following steps:
Create a new function called
run
, which takesraw_data
andrequest_headers
as parameters and returns a dictionary of results as follows:Copy the code under the 'Prepare Data' and 'Score Data' headings into the
run
function.The
run
function should look like the following code (Remember to remove the statements that set the variablesraw_data
andrequest_headers
, which will be used later when therun
function is called):
Once the run
function has been created, replace all the code under the 'Prepare Data' and 'Score Data' headings with the following code:
The previous code sets variables raw_data
and request_header
, calls the run
function with raw_data
and request_header
, and prints the predictions.
After refactoring, experimentation/Diabetes Ridge Regression Scoring.ipynb
should look like the following code without the markdown:
Combine related functions in Python files
Third, related functions need to be merged into Python files to better help code reuse. In this section, you'll be creating Python files for the following notebooks:
- The Diabetes Ridge Regression Training notebook(
experimentation/Diabetes Ridge Regression Training.ipynb
) - The Diabetes Ridge Regression Scoring notebook(
experimentation/Diabetes Ridge Regression Scoring.ipynb
)
Create Python file for the Diabetes Ridge Regression Training notebook
Convert your notebook to an executable script by running the following statement in a command prompt, which uses the nbconvert
package and the path of experimentation/Diabetes Ridge Regression Training.ipynb
:
Once the notebook has been converted to train.py
, remove any unwanted comments. Replace the call to main()
at the end of the file with a conditional invocation like the following code:
Your train.py
file should look like the following code:
train.py
can now be invoked from a terminal by running python train.py
.The functions from train.py
can also be called from other files.
The train_aml.py
file found in the diabetes_regression/training
directory in the MLOpsPython repository calls the functions defined in train.py
in the context of an Azure Machine Learning experiment run. The functions can also be called in unit tests, covered later in this guide.
Create Python file for the Diabetes Ridge Regression Scoring notebook
Covert your notebook to an executable script by running the following statement in a command prompt that which uses the nbconvert
package and the path of experimentation/Diabetes Ridge Regression Scoring.ipynb
:
Once the notebook has been converted to score.py
, remove any unwanted comments. Your score.py
file should look like the following code:
The model
variable needs to be global so that it's visible throughout the script. Add the following statement at the beginning of the init
function:
After adding the previous statement, the init
function should look like the following code:
Create unit tests for each Python file
Fourth, create unit tests for your Python functions. Unit tests protect code against functional regressions and make it easier to maintain. In this section, you'll be creating unit tests for the functions in train.py
.
train.py
contains multiple functions, but we'll only create a single unit test for the train_model
function using the Pytest framework in this tutorial. Pytest isn't the only Python unit testing framework, but it's one of the most commonly used. For more information, visit Pytest.
A unit test usually contains three main actions:
- Arrange object - creating and setting up necessary objects
- Act on an object
- Assert what is expected
The unit test will call train_model
with some hard-coded data and arguments, and validate that train_model
acted as expected by using the resulting trained model to make a prediction and comparing that prediction to an expected value.
Next steps
Now that you understand how to convert from an experiment to production code, see the following links for more information and next steps:
Import Markdown Python Example
- MLOpsPython: Build a CI/CD pipeline to train, evaluate and deploy your own model using Azure Pipelines and Azure Machine Learning
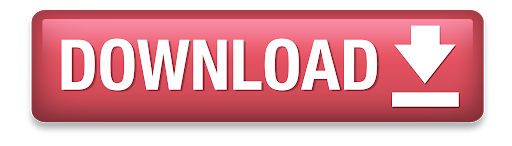